The IADGENOL project deals with the fields of AWES (airborne wind energy systems) technologies and deep learning and artificial intelligence. The goal of this project was to research and apply automatic control of AWES systems through machine learning using data obtained both from a simulation environment and from an operating AWES machine.
AWES represent an innovative approach to harnessing wind energy from higher layers of the atmosphere, but it faces significant challenges, especially regarding operational autonomy. Currently, achieving robust autonomous control is one of the main obstacles; systems must be fully autonomous and withstand varying weather conditions over long periods. To improve the adaptability of traditional control systems, the use of machine learning and reinforcement learning has been explored.
The main objective of the IADGENOL initiative was to create a deep learning-based model to automatically control the trajectory of AWE systems, as well as to use these models to understand and characterize the dynamic challenges faced by such systems. This project was carried out entirely by CT using its own resources and the shared AWES test machine, available through a collaboration agreement with Madrid’s Carlos III University to develop AWES technologies. The tasks performed ranged from preliminary research on the state of the art, data mining and processing to use in the the model, to the actual development of data-driven models and the controller itself.
Validation tests of the control system were conducted in real-world operation. Prior to these tests, the controller was trained in an AWES simulation environment developed by CT. The results of this test, simulating a “natural learning” process, were very promising. It showed that the kite was able to align with the wind and perform figure-eight patterns—trajectories typically used in such systems to maximize energy generation.
On the other hand, the transition from the simulated environment to real-world implementation was not entirely successful. However, due to the encouraging results obtained during simulations, it is believed that the issue could be resolved by refining the simulation methods and creating controlled laboratory environments that allow these algorithms to be trained with minimal risk.
Main results:
During the execution of the project, three parallel research lines were developed: a deep learning-based controller, a system for characterizing the physics of an AWES system, and a wind prediction system.
For the deep learning-based controller:
A controller was developed using deep reinforcement learning (DRL) algorithms, ultimately selecting the one based on TD3 (twin-delayed deep deterministic policy gradient).
The controller demonstrated stable performance in guiding the kite through figure-eight trajectories in a simulated environment without wind gusts, although its effectiveness was limited by variable winds due to simulator constraints. Later, the controller was integrated with the control system of the real AWES machine and tested under real conditions. Although efficient kite control was not achieved—due to the lack of training in a real environment and associated difficulties—the controller’s proper integration with the machine was successfully verified.
Physics Characterization System:
Dynamic models based on neural networks were developed to predict the flight dynamics of the system. These models proved effective in predicting dynamics over a 0.1-second time horizon. However, error propagation in the predictions affected the system’s performance compared to a reference trajectory.
Wind Prediction System:
A system was developed to predict wind at different altitudes using surface meteorological data. However, the accuracy evaluation of the predictions was limited due to the lack of adequate measuring equipment. Despite this, the system was successfully integrated into the controller, enabling real-time decision-making.

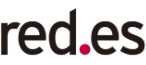
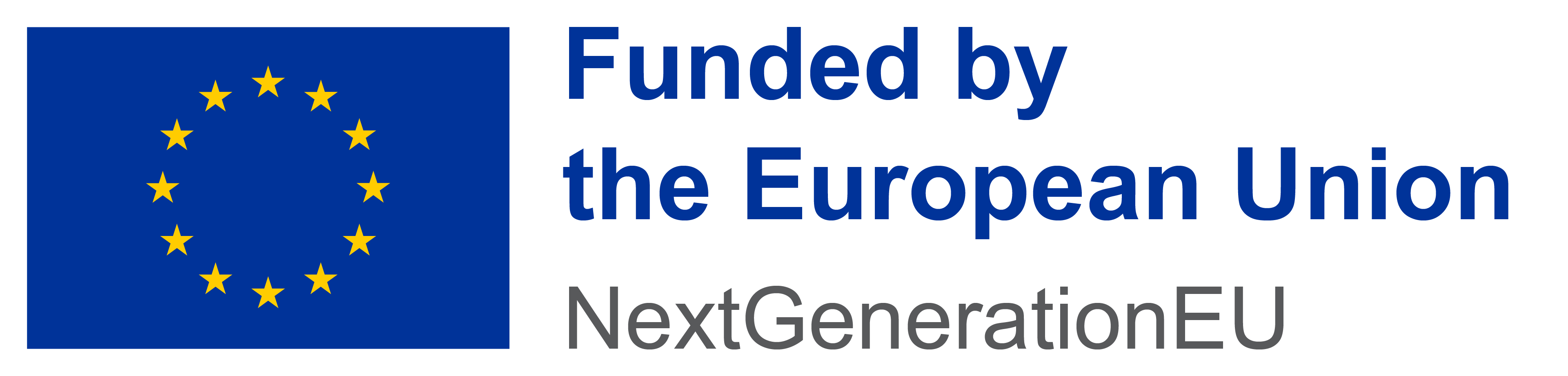
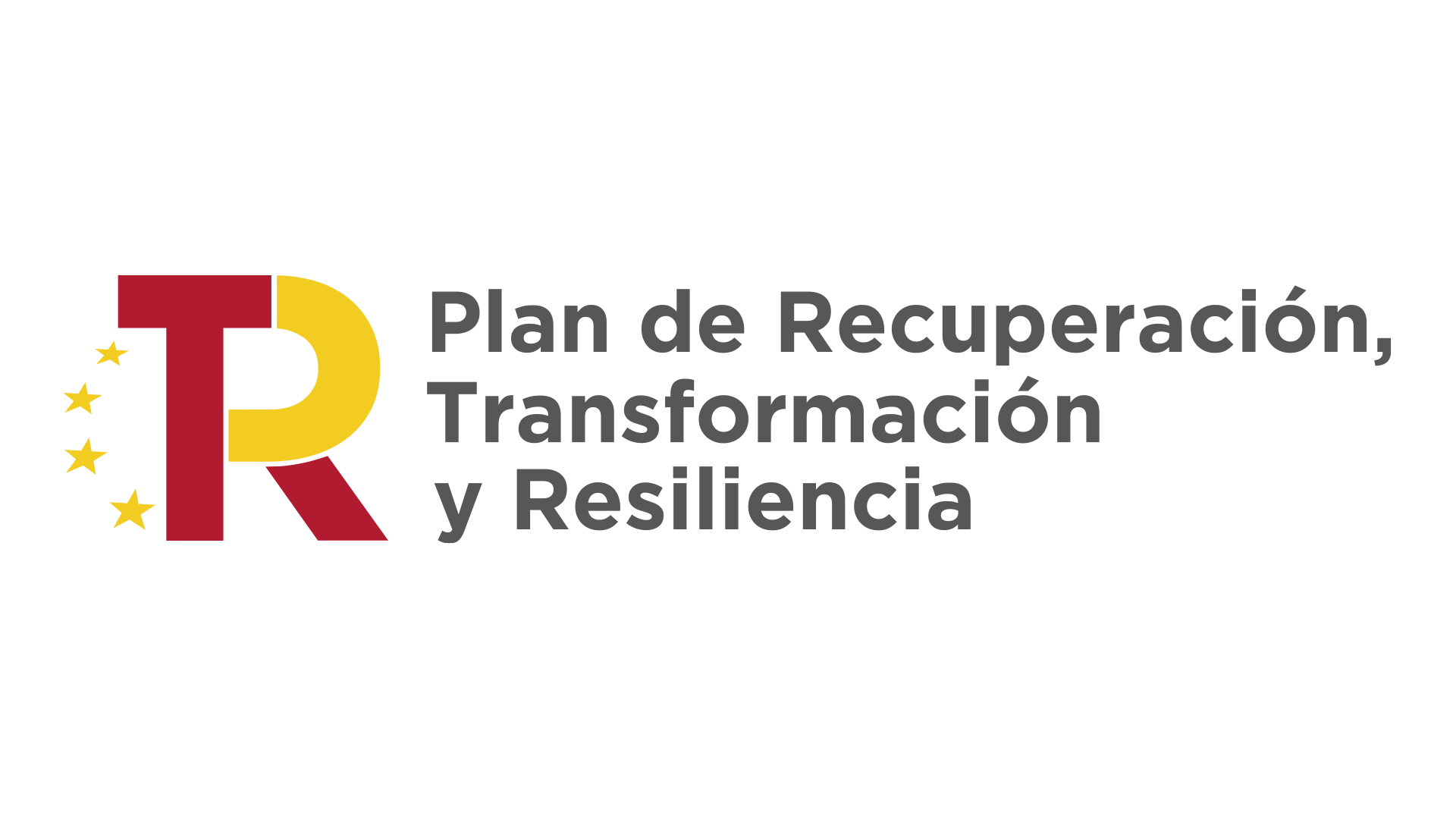